Show:
Data Science Engineering Services: Transforming Data Into Insights
In the rapidly evolving digital landscape, data is the fuel that powers innovation and decision-making. Data science engineering services play a pivotal role in transforming raw data into actionable insights.
By leveraging advanced techniques and machine learning algorithms, businesses can unlock the full potential of their data. This article explores the importance of data science engineering, the key steps involved in data transformation, and the techniques for extracting valuable insights.
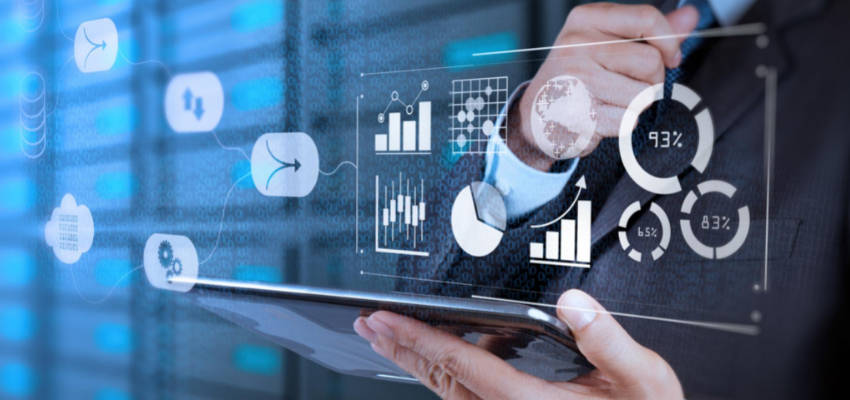
Discover how data science engineering services can drive business growth and enable informed decision-making.
The Importance of Data Science Engineering
Before diving into the significance of data science engineering services, let’s understand how they can empower organizations to make data-driven decisions and boost their overall business performance. Data science engineering’s importance lies in its ability to leverage data to drive strategic decision-making and enhance business performance. With the increasing volume, velocity, and variety of data generated by organizations, it has become crucial to implement data engineering best practices to ensure efficient data processing and analysis.
Data engineering involves the collection, transformation, and storage of data, enabling organizations to derive meaningful insights and make data-driven decisions. By applying data engineering best practices, businesses can ensure data quality, integrity, and security, enabling accurate analysis and decision-making.
Data-driven decision making allows organizations to identify patterns, trends, and correlations within the data, enabling them to make informed decisions, optimize processes, and discover new opportunities.
Key Steps in Data Transformation
To successfully transform data and extract valuable insights, certain key steps must be followed in the data transformation process.
One of the crucial steps is data cleaning, which involves handling missing values, outliers, and inconsistencies in the dataset. This process ensures that the data is accurate and reliable for analysis.
Another important step is feature engineering, which involves creating new features or transforming existing ones to improve the predictive power of the data. This can include techniques such as encoding categorical variables, scaling numerical features, or generating interaction terms. Feature engineering helps capture the underlying patterns and relationships in the data, leading to better predictive models.
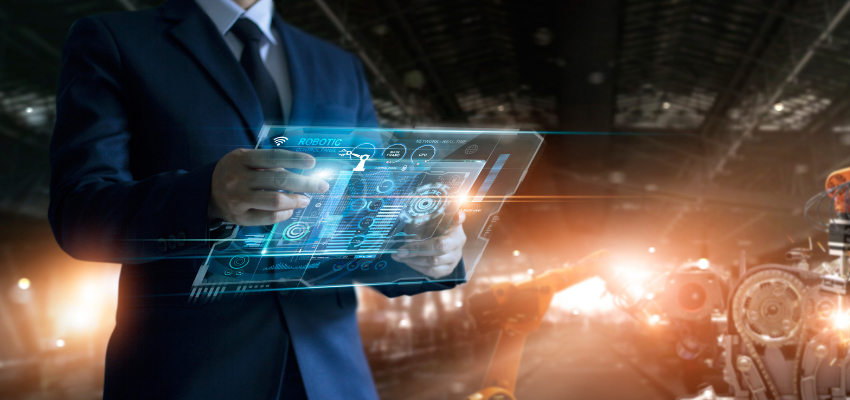
Techniques for Extracting Insights
One essential aspect of data science engineering services is employing various techniques to extract valuable insights from the transformed data. Data visualization techniques and data mining methods are two commonly used approaches in this process.
Data visualization techniques involve visualizing the data in a graphical format to identify patterns, trends, and anomalies. By presenting the data in a visual form, analysts can easily interpret and understand complex relationships within the data. This helps in making informed decisions and discovering actionable insights.
On the other hand, data mining methods involve applying statistical algorithms and machine learning techniques to identify patterns and relationships in large datasets. These methods help in uncovering hidden insights, predicting future trends, and making data-driven decisions.
Leveraging Machine Learning Algorithms
Employing machine learning algorithms is a crucial step in leveraging the transformed data to extract valuable insights in data science and engineering services.
Machine learning algorithms automate the process of analyzing data and making predictions. These algorithms use statistical techniques to identify patterns and relationships within the data, allowing for predictive modeling.
By training these algorithms on historical data, they can learn to make accurate predictions on new, unseen data. This automation of processes enables data scientists to efficiently analyze large volumes of data and generate insights quickly.
Predictive modeling, a key application of machine learning, allows organizations to forecast future trends and make data-driven decisions.
Leveraging machine learning algorithms in data science engineering services can significantly enhance the efficiency and accuracy of data analysis, enabling businesses to gain a competitive edge.
Driving Business Growth With Data Science
Data science is a catalyst for driving business growth. By harnessing the power of data, organizations can gain valuable insights and make informed decisions to propel their success.
One way data science drives business growth is through the use of business intelligence. By analyzing large volumes of data, businesses can uncover patterns, trends, and correlations that help them understand customer behavior, market dynamics, and competitive landscapes. This knowledge enables businesses to optimize their strategies and operations, resulting in increased efficiency, productivity, and profitability.
Predictive analytics plays a crucial role in driving business growth. By leveraging advanced algorithms and statistical models, organizations can forecast future trends, anticipate customer needs, and identify new opportunities, enabling them to stay ahead of the competition and make data-driven decisions that fuel business growth.